Infrastructure enhancements targeting AI workloads include updates to compute hardware, new Nvidia GPU offerings, and storage optimization.
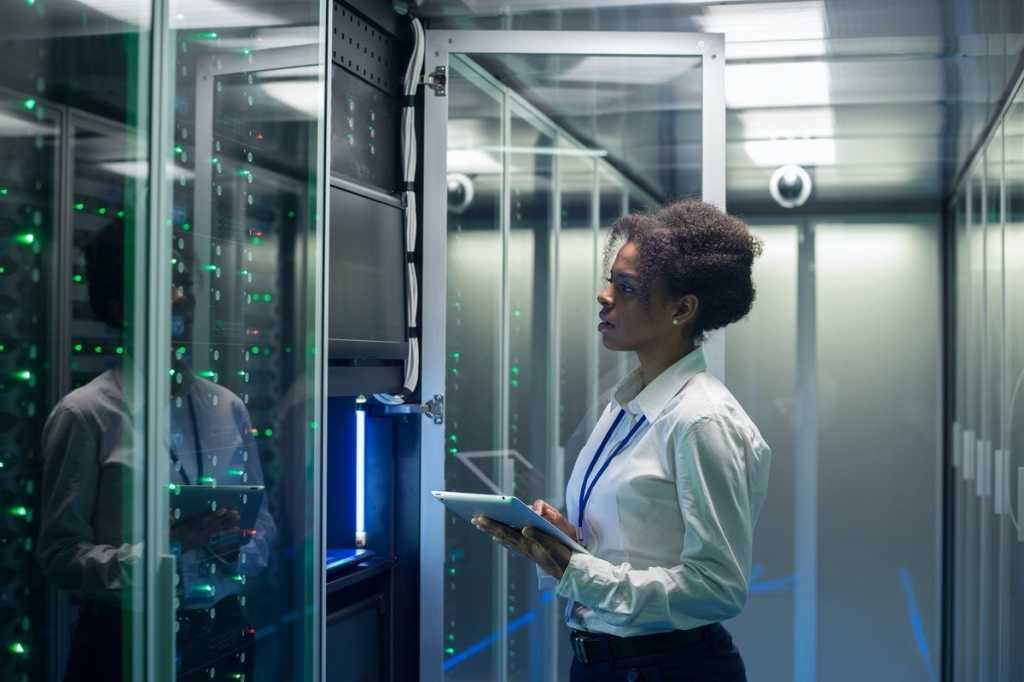
Google has showcased a range of updates to its cloud infrastructure at its annual Cloud Next conference, the better to support AI workloads and help enterprises optimize cloud expenditure.
Updates include are faster processors, bigger virtual machines, more storage, and new management tools.
First up: Google has made the latest iteration of its proprietary accelerator module for AI workloads, the Tensor Processing Unit (TPU) v5p, generally available in its cloud.
A single TPU v5p pod contains 8,960 chips that run in unison, over twice as many as in a TPU v4 pod, the company said, adding that it also delivers over twice as many flops and three times the high-bandwidth memory per chip.
The TPU pods now have support for Google Kubernetes Engine (GKE) and multi-host serving on GKE: “TPU multi-host serving on GKE allows customers to manage a group of model servers deployed over multiple hosts as a single logical unit, enabling users to manage and monitor them centrally,” Google said.
The TPU isn’t the only new hardware addition. Under an expanded partnership with Nvidia, Google is also introducing the A3 Mega virtual machine (VM) to its cloud, powered by Nvidia H100 GPUs.
It was in May 2023 that Google first launched the A3 series of supercomputer VMs in its cloud, aimed at rapidly training large AI models.
The new A3 Mega VM, which will be generally available next month, offers double the GPU-to-GPU networking bandwidth of the original A3, the company said, adding that it was planning to add Confidential Computing capabilities to the A3 VM family in preview later this year. The feature is intended to protect the privacy and integrity of data being used in AI workloads.
Storage optimization for AI and ML workloads
To improve performance on AI training, fine-tuning, and inference, Google Cloud has made enhancements to its storage products, including caching, which keeps the data closer to compute instances and enables a faster training cycle.
The enhancements are targeted at maximizing GPU and TPU utilization, leading to higher energy efficiency and cost optimization, the company said.
One of these enhancements is including caching in Parallelstore, a managed parallel file service that offers high performance. While this enhancement is still in preview, it can offer up to 3.9 times faster training times and up to 3.7 times higher training throughput compared to native ML framework data loaders, the company said.
Another enhancement is the introduction of a preview of Hyperdisk ML, a block storage service optimized for AI inferencing workloads.
“It accelerates model load times up to 12X compared to common alternatives, and offers cost efficiency through read-only, multi-attach, and thin provisioning,” the company said.
It enables up to 2,500 instances to access the same volume and delivers up to 1.2 TiB/s of aggregate throughput per volume, which according to Google is over 100 times greater performance than Microsoft Azure Ultra SSD or Amazon EBS io2 BlockExpress.
Other storage changes includes the general availability of Cloud Storage FUSE, a file-based interface for Google Cloud Storage (GCS) targeted at complex AI and ML applications, and Filestore, which is optimized for AI and ML models that require low latency, file-based data access.
“Filestore’s network file system-based approach allows all GPUs and TPUs within a cluster to simultaneously access the same data, which improves training times by up to 56%,” the company said.
New resource management and job scheduling service
To help enterprises optimize costs, Google Cloud is also adding a resource management and job scheduling service for AI workloads, named the Dynamic Workload Scheduler.
This improves access to AI computing capacity and helps enterprises optimize their spend for AI workloads by scheduling all the accelerators needed simultaneously, and for a guaranteed duration, the company said.
Dynamic Workload Scheduler offers two modes — flex start mode for enhanced obtainability with optimized economics, and calendar mode for predictable job start times and durations.
While the flex start mode is used to queue AI tasks that need to run as soon as possible on the basis of resource availability, the calendar mode offers short-term reserved access to AI-optimized computing capacity.
Both the modes are currently in preview.